Non-Performing Loans
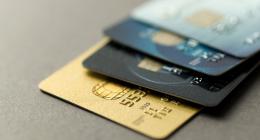
Reducing costs and diversity on Maintenance Repair & Overhaul (MRO) parts is very challenging.
Reducing costs and diversity on Maintenance Repair & Overhaul (MRO) parts is very challenging. Whilst Procurement is intending to reduce cost (and diversity) through preconfigured catalogues, Maintenance is requiring full availability of parts, in order not to disrupt production lines.
Automotive and Aeronautic manufacturers are managing thousands of references & suppliers, on dozens of production sites. Executing MRO purchasing strategies requires online catalogs embedded with Sourcing to Pay solutions, but can’t we go one step further? Since Procurement strategies are more and more data driven, how could data science contribute to more sophisticated and efficient levers?
Data science tools, such as Natural Language Processing (NLP), can drastically accelerate data cleaning, anomalies detection, references matching. Thanks to data science, heterogeneity of references can be slowed down by pointing out overlaps, redundancies of references.
Procurement teams have access to three types of data:
Considering the volume of data (tens of thousands of references), automation is required to clean and process data: reconciliation of these 3 databases into a single and clean source of information is very valuable for companies. Matching items by their respective references across these three databases is challenging due to unstructured data . NLP techniques (such as string pattern recognition or Levenshtein distances) applied on item descriptions enable the matching of items belonging to two or three databases. Every item gets a reference number shared on every database.
Thanks to this new work frame each purchased item can have its invoiced price compared to the negotiated price (prices compliance process). “Public” prices can also be massively compared to negotiated prices (price competitiveness process). Significant discrepancies in comparison with market prices are therefore pointed out.
For instance, applying the price compliance process on 1,400+ orders, an unjustified extra cost of over 4% has been identified. Extended analysis can then easily spot the overpriced items to perform deep dives.
80% of these 350 references are bought at the expected price or below. However, 20% of the orders present overpriced items corresponding to 4% of the spend.
Most items of this specific supplier have a discount of 30%+ over the public prices found on the internet. Some items however have only small or no discount. Data science analysis helps the procurement team to spot them.
Price competitiveness has been studied for 5 suppliers. For each one of them an anomaly detection algorithm has been used to spot references with an unjustified high price compared to both other references and public. Procurement analysts can then check these specific references to make sure each price is relevant.
Thanks to data capture, the procurement team have an updated knowledge on the suppliers ‘products, prices, availability and detailed description. Data cleaning and database matching techniques make this new source of information highly valuable. The Procurement team can point out potential savings and share these opportunities with stakeholders (and key users).
Furthermore, these structured data bases strongly contribute to streamline the procurement processes. From the new structured data, algorithms can detect items that are purchased from two or more suppliers, which leads to a potential reduction of the supplier panel. NLP techniques on item descriptions can spot those which are similar or even exchangeable. Key users can then focus on these specific items to reduce the number of purchased references, reduce diversity and improve process efficiency.
As a starting point, business use cases need to be identified, usually resulting from key operational dysfunctions. The question could be “what could we significantly improve …, if only we had this kind of analysis”. Then, prepare the set of data on a limited scope and share this with data scientists in a collaborative approach to evaluate feasibility.
The test will then consist into realizing of a first Proof of Concept over a few weeks to confirm the feasibility and measure the expected impacts, leading into a Business Case.