Canadian Hydrogen Observatory: Insights to fuel…
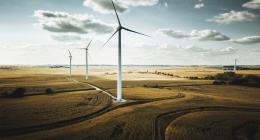
Rethinking privacy in the AI era
Concerns over data privacy have peaked in recent years, largely in tandem with the increased use of advanced technologies for data processing and collection, like artificial intelligence. Roughly 80% and 60% of Americans respectively report feeling a lack of control over their data collection and a lack of understanding about their data use [1]. This is unsurprising given the recent timeline of privacy-impacting events. In 2010, we saw Edward Snowden’s revelations about the US government surveillance tactics and their effect on the EU-US Safe Harbor Framework, in 2017, the Equifax breach of American credit score data and in 2018, the Cambridge Analytica scandal’s escalation to the forefront questions about unfettered data transfers to third parties.
Today, in a time of the COVID-19 pandemic, when spread of disease foments death and economic depredation, our concerns over privacy remain centerstage. As nations manipulate their data practices to fight the pandemic, there is a tangible rise in concern over privacy preservation in the use of contact tracing, antibody passports, migration to work from home environments, temperature / symptom logs with return to offices, and in minors’ data as they increase use of video streaming platforms like TikTok. In the clout of these events that leave us reactive to privacy implications, Sia Partners turns our attention to two questions: (1) how can we preserve privacy while promoting the use of AI and (2) how can we use AI to better predict and prepare for events impacting our privacy compliance.
In an age where the pace of technological innovation is simply too fast for the law to follow, it has become necessary to consider the following – how can businesses remain privacy compliant in the age of artificial intelligence?
The U.S.’s federal Future of Artificial Intelligence Act (2017) and the U.K. Information Commissioner’s Office (“ICO”)’s final guidance on Artificial Intelligence (the “Guidance”) (2020) sought to take the first steps in privacy preservation against potential abuse through the use of AI. The key takeaway from both the U.S. and U.K. frameworks is a familiar one: identification and mitigation of privacy risks at the design stage is likely to yield the most privacy-preserving compliance (“privacy by design”). Indeed, we observe businesses moving away from the former reactive models of retrofitting privacy consciousness at the end of a project or product lifecycle. Instead, companies try to address privacy issues at early stages of each project – including AI initiatives – protecting the consumer from potential discrimination, lack of transparency, and data abuse. To do so, a few central principles should be respected: [2]
As businesses increase integration of privacy by design principles, we are observing both a regulatory and business evolution of privacy compliance techniques. Our privacy journeys today have taught us that 2018’s recommendations for encryption and anonymization of data alone are not enough to preserve the security and privacy of underlying personal data. Consequently, our approach has evolved and we see organizations considering the application of privacy-enhancing techniques such as perturbation, federated learning, differential privacy, homomorphic encryption, secure multi-party computation, and the use of synthetic data to training data to minimize the risk of data linkages for identification of individuals. In this vain and in moving forward, organizations should consider how might technology and innovation themselves step in and help the privacy-consciousness of AI systems
Despite the increased acceptance of privacy by design tenets by the leading innovators of the world, unforeseen events such as the Cambridge Analytica scandal and COVID-19 have left businesses reacting to the unpredictability of privacy implications. Naturally, the timeline of privacy events sparks a curiosity about the potential to leverage data itself, through AI, to enhance, supplement, and even succeed the role of business in making accurate predictions. These predictive abilities could be modified to enhance consumer controls, monitor the regulatory landscape, update clauses in third party contracts, and enhance data quality and security controls. That is, rather than trying to account for privacy compliance through governance models and lengthy disclosures, AI can be used to ingrain privacy protections into data architectures to be maintained partially, or fully, automatically. This translates to enhanced control for organization’s over their privacy obligations through using predictive models focused both on the privacy preferences of consumers and on their business frameworks.
What does this look like? This appears as seamless privacy notice and choice systems where AI can be used to exchange computer-readable privacy policies and user-consent statements between a consumer-preference focused AI system and the devices and services with which the user’s data interacts. The consumer-preference focused AI system would intelligently learn the privacy preferences of users over time to predict the consumer’s true preferences regarding their personal data processing and purpose. This would semi-automatically configure many settings, making privacy decisions on behalf of the end-consumer.
Why is this valuable? Studies show that consumers’ actual attitudes towards their preferences on their own data collection is variable dependent on the time of day and on what they have recently heard, seen, or read [3]. Further, consumers often do not want to be bothered by choice at the time of consent acquisition or misunderstand the disclosures – a use case held to be illegitimate consent acquisition under the EDPB’s guidance on “click fatigue” May 2020 [4]. Given the incredible complexities of legitimate consent acquisition, it makes sense to look to predictive AI models to help – to integrate into browsers and train them and operating systems to choose privacy controls based on the user’s learned preferences.
What does this mean for business? For business, this means partially automated privacy compliance. In this or similar models, personal data of end consumers is not collected or processed until legitimate consent is acquired and matched to the user’s designated preferences. An audit trail of all data flows and lineage across systems and parties is available to users and companies. Based on predictive learning models focused on consumer privacy preferences, the room for innovation in advertising technology is limitless – people who have lawfully consented to be alerted to new products can receive ads precisely targeted to the product, service, time, and location best suited to the interests of the end-consumer. In other words, this model uses AI / ML to aid the user in managing one’s personal privacy choices and in turn, opens the flow of data for the promotion of business development.
While efforts in sophisticating our current technologies are being piloted, digital assistance for consumer preferences is certainly a target in global privacy compliance, and one whose development we monitor closely. More immediately, our current state of AI capabilities – focused on the aims of business – can be repurposed to become more predictive for larger privacy compliance. Among these tasks would be to foresee possible actions of regulators in response to use cases, monitor the ever-changing privacy regulatory landscape and assign risk ratings specific to an organization, identify and update contractual clauses in third party contracts, identify and classify personal data within systems, identify and remove points of human intervention over the lifecycle of sensitive data, enhance data quality and accuracy, reduce algorithm bias, and enhance security controls over systems and underlying data.
In both models, it can be suggested that viewing privacy as a space for competitive differentiation and technological innovation (enhanced brand reputation and consumer reach) allows privacy to shift from advocacy of policy (too often trailing technological development) towards a method of operationalizing capabilities through technology. With cumbersome privacy disclosures aimed at preventing litigation rather than at preserving privacy and in a world where it is invariably impossible to live outside the digital ecosystem, robotic assistance to help consumers and business navigate dubiety certainly sounds appealing.
“We understand the benefits that AI can bring to organizations and individuals, but there are risks too. That’s why AI is one of our top three strategic priorities [ . . . ] It is important that you do not underestimate the initial and ongoing level of investment of resources and effort that is required. Your governance and risk management capabilities need to be proportionate to your use of AI. This is particularly true now while AI adoption is still in its initial stages, and the technology itself, as well as the associated laws, regulations, governance, and risk management best practices are still developing quickly.” – ICO the “Guidance” on Artificial Intelligence, July 2020
With 100+ projects delivered, Sia Partners has extensive experience helping companies reinforce their Privacy Policies, Procedures, and Standards. Additionally, our Centers of Excellence have developed technical AI solutions to support the maturity of your organization’s Privacy Program.
David Gallet
Associate Partner
(347) 577 2063
Manager
(917) 442 3527
Supervising Sr. Consultant
(732) 841 2679
References:
1. “Americans and Privacy: Concerned, Confused, and Feeling Lack of Control Over Their Personal Information.” Pew Research Center: Internet, Science & Tech, Pew Research Center, 19 Nov. 2019
2. “Guidance on AI and Data Protection.” ICO, July 20
3. Acquisti, Alessandro, et al. What Is Privacy Worth? 2013
4. Guidelines 05/2020 on Consent under Regulation 2016/679