Canadian Hydrogen Observatory: Insights to fuel…
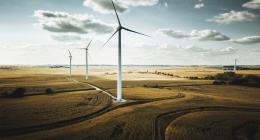
Data scientists can play a key role in the organization of a Distributed System Operator (DSO). Amidst a tsunami of data, facilitated by an increasing intelligent distribution grid, data scientists are able to create value from raw data.
Equipped with an extensive skillset, data scientists can catalyze operational excellence and develop new business models. However, to enable these professionals, DSO's first need to fulfill a handful of conditions to prevent data scientists from finding themselves stuck between different layers in the organization.
Following the Big Data revolution, many companies have opened up positions for data scientists to join their organization. The definition of a data scientist differs, but there is consensus on his/her role in general. A data scientist helps organizations to maximize value from data originating from customers, suppliers, internal research and other (open) data (initiatives). Specific to the Distribution System Operator (DSO), there are both external and internal factors which require “intelligent data solutions”. A data scientist is a key actor for shaping these solutions.
General trends and challenges for the DSO :
In this article, the role of a data scientist at a DSO will be highlighted. What is the skillset of a data scientist? What environment should a DSO offer to enable a data scientist? We also present some specific cases for a DSO where the data scientist can add value.
Traditional Business Intelligence (BI) departments are not able to provide proper “intelligence” in the world of big data. Whereas traditional BI is primarily question-driven (i.e. managed top-down), data scientists often act in a more vague arena. In general, BI[1] focusses on relatively straightforward problems by querying and/or utilizing data in a structured form. Data scientists however should be able to truly find structure in chaos, using non-structured data from all kinds of sources to bridge the gap between (data) management and analysis. In order to fulfill such needs, a data scientist not only requires a toolbox full of hard skills, such as machine learning and statistics, but also domain knowledge and communication skills (see figure 1). Above all, data scientists should have a pro-active, entrepreneurial and autodidactic mindset in order to identify business problems and continuously sharpen their skillset. In summary, a data scientist should have multidisciplinary skills and the drive to ‘get to the bottom’. A huge demand for data scientists is expected over the coming years.
Figure 1. The extensive skillset that enable data scientists to answer some of the most complex and big-data-driven management questions
Data scientists are no magicians, they need to work in the right conditions in order to prosper in the organization. In case an organization fails to fulfill a number of basic conditions, hiring a data scientist is just money down the drain. Most importantly, an organization should first get its (big) data under control. This means that there should be an overarching enterprise architecture (EA) and basic data governance framework already implemented. The objective is to make data accessible from multiple sources and to assure data quality.
Using that starting point, a data value chain can be set up, depending on the management question(s) to be answered. In principle, such a value chain consists of 3 elements (see figure 2). First, it starts by opening up data sources in order to be able to access and relate the data. Scattered and overlapping systems undermine the productivity of a data scientist. For example DSO’s should connect their systems for:
In the second step of the chain, the data scientist puts the data to work. Starting with simple models, rapid prototyping and testing hypotheses, a data scientist moves towards more complex simulation and modelling if needed.
In the final step of the chain, the outcomes of the data processing step are made accessible and transparent by visualization or by making it available for systems further down the chain.
Figure 2. The basic building blocks of an example data value chain, from data sourcing to data processing and modelling to data visualization. From a system’s perspective, a data scientist should be enabled by setting up a data architecture and management. This secures accessibility and quality of data before a data scientist can really add value to it.
There are many examples for DSO’s where data scientists can add value to the problem solving process. Some of the three main DSO activities are taken as an umbrella in the example cases below :
Asset management
Congestion management
Maintenance engineering
This article displayed the role and “factors of enablement” for a data scientist in a DSO context. The key question now is how to convert this potential to actual value in the day-to-day activities. Although data science is still a relatively new phenomenon, DSO’s should adopt a pro-active approach to embed it in their organization. There should be however a clear link with the overall company strategy – especially the digital strategy – to ensure that effective and value-add (cascaded) activities are deployed by the data science department.
At Sia Partners, we believe that the inclusion of data should play a more important role in the decision making process, especially now that the grid becomes ‘smarter’. In this new data era, the processing of an extreme high volume and velocity of data will be the new norm. Without properly embedding the data science department in the organization, DSO’s will fail to make the most optimal decisions. Both for their long-term strategic decisions like on investment planning and short-term operational decisions like congestion management.
As stipulated in the Dutch Energy Agreement (“Nationaal Energieakkoord”) DSO’s are making a first step by promoting and driving data disclosure in open data initiatives. This shows that DSO’s are already making a change and becoming more data-aware.
At Sia Partners, we help companies to set up their digital agenda including the correct application and implementation of a data science hub.
[1] In this article, Business Intelligence and Data Science are generalized according to the theory. However, in practice, the distinction between both worlds is sometimes less clear.