CrowdStrike Falcon BSOD Issue: Troubleshooting…
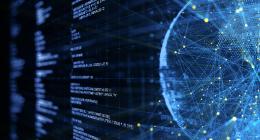
Collecting and exploiting quantitative data to define strategic areas for day-care openings
Location intelligence is the combination of location data and any form of business intelligence that helps companies get additional insights through spatial analyses. It allows for the creation of interactive maps highlighting the relationships between different metrics in a physical space and can be combined with external data such as economic growth and demographics. Many business challenges can be addressed through location intelligence, such as geographic marketing, network planning and design, flow optimisation or retail modelling.
Similarly, site planning – the process of optimising where to open its next location - is a challenge for every company operating a brick and mortar store, or every public service agency offering a physical location. Most entities need to rely on costly and lengthy market research, which can become complicated if many new locations are needed. Since this research is mostly based on quantitative elements, how could data science - through the use of location intelligence - contribute to the automation and analysis of this important issue?
Factors that are routinely used to determine a sector’s overall attractiveness can be economic, socio-demographic, or based on the proximity of potential competitors. By combining the three and finding ways to automate certain processes, it becomes possible to pinpoint specific locations to recommend. This is what we achieve with the « location intelligence for day-cares bot», where we took day-cares as the industry to analyse.
In France, finding a day-care for a child can be a strenuous task: waiting lists are often long. For example, the number of available places in 2018 was at 56.6 per 100 children under 3 years old. Additionally, there are significant territorial disparities, with a capacity that varies from 10 places in French Guiana to nearly 92 places (per 100 children) in Haute-Loire. Paris and the Hauts-de-Seine have the highest capacity, with 67 and 63 places for 100 children under 3 years old. This disparity in supply and demand makes it interesting for new day-cares to enter the market.
With this bot, the goal was to create an interactive environment where users could visualise potentially attractive areas for new locations. To achieve this as a proof of concept, the perimeter was restricted to the city of Bordeaux, but can be easily extended to any other territory.
The data required for this bot is obtained from various sources: existing day-care names, locations, and the information on the quantity of available places is captured through web scraping, while economic and socio-demographic data is retrieved through open data sites hosted by government institutions. The crossing of these different sources of data creates an important and original basis on which most of the following work can be achieved.
The analyses that mainly concerned geo-spatial data calculations and visualisations were all done using a PostGis database and a geo server, which allowed the creation of a hexagonal grid on the geographical area of Bordeaux. This technique was employed because of the need for smaller areas than the ones defined by Iris, particularly in regards to scoring. Recommending an area that is too large would not be beneficial for companies that are generally looking at more specific areas like streets or street corners. With the city divided into these new areas, economic and socio-demographic statistics were calculated on each new zone, serving as input variables for the different scores.
Three specific scores were created in order to quantify each zone’s attractiveness for the opening of a new day-care:
Finally, the Global score is derived from a mathematical formula that takes the supply/demand score and the economic score to calculate the overall potential appeal of an area.
Leveraging location intelligence for day-cares
Through assignments and projects, Sia Partners developed a strong expertise in exploiting and leveraging territorial data that relates to location intelligence . Some of our use-cases are showcased on our online Data Science Showroom. In the «Move’s impact on daily commute time» bot, an application was created that calculates the impact of travel time on employees’ daily commute in the event of a firm’s relocation. Many of the same concepts as the « location intelligence for day-cares bot» are used, including the mapping of possible new office locations and employees’ addresses, distance and length of travel time calculations, and the creation of a visual dashboard displaying different results based on the analyses.
Similarly, the « Forecast availability bot » maps parking spaces and allows users to visualize forecasts of occupancy rates calculated using historical data, weather forecasts, nearby events, and traffic data.
The key takeaway from all these projects is that true insights can be drawn when geographical data is crossed with other types of data (time-series data, market data, socio-economic data, weather data, etc.)
The merging of informational and geographical data allows numerous opportunities for private and public entities. Thanks to the availability of socio-demographic information on government open data websites, any private or proprietary data can be augmented by analysing it within its socio-demographic or economic context. For example, a bank wishing to optimise its ATM network can use customers' usage patterns, combined with scraped data on its competitors' locations and open data on populations to create an optomisation problem. Results can then be used to determine the best areas in which to place their ATMs, or to map the results in order to create a visual tool similar to this one.
Franchisers can also use this technology to locate strategic new locations based on the spatial join of internal data about consumption patterns at their current franchisees, current franchisee locations, competitor locations, and socio-economic data applied by area. Furthermore, this could easily be converted into a supervised problem given the availability of internal data such as revenue. The aforementioned variable would serve as the dependent variable, while all other explanatory variables that vary per location would be used in model fitting. This model would be applied to all new areas in order to generate a predicted revenue, which could then be used to generate a score. Given the advancements in prediction performance due to models such as boosting or neural networks, transforming this problem into a supervised one could achieve interesting results.
Evidently, these overall strategies are very similar to the one laid out for the bot, showing that the general idea can easily be replicated to suit a number of different business problems.
[1] La CAF
[2] Iris is the geographic unit for dissemination of infra-municipal data in France. These units must respect geographic and demographic criteria and have borders which are clearly identifiable and stable in the long term. Towns with more than 10,000 inhabitants, and a large proportion of towns with between 5,000 and 10,000 inhabitants, are divided into several IRIS units. This separation represents a division of the territory. France is composed of around 16,000 IRIS of which 650 are in the overseas departments. By extension, in order to cover the whole country, all towns not divided into IRIS units constitute IRIS units in themselves.
If you want to know more about our AI solutions, check out our Heka website
Contact : david.martineau@sia-partners.com